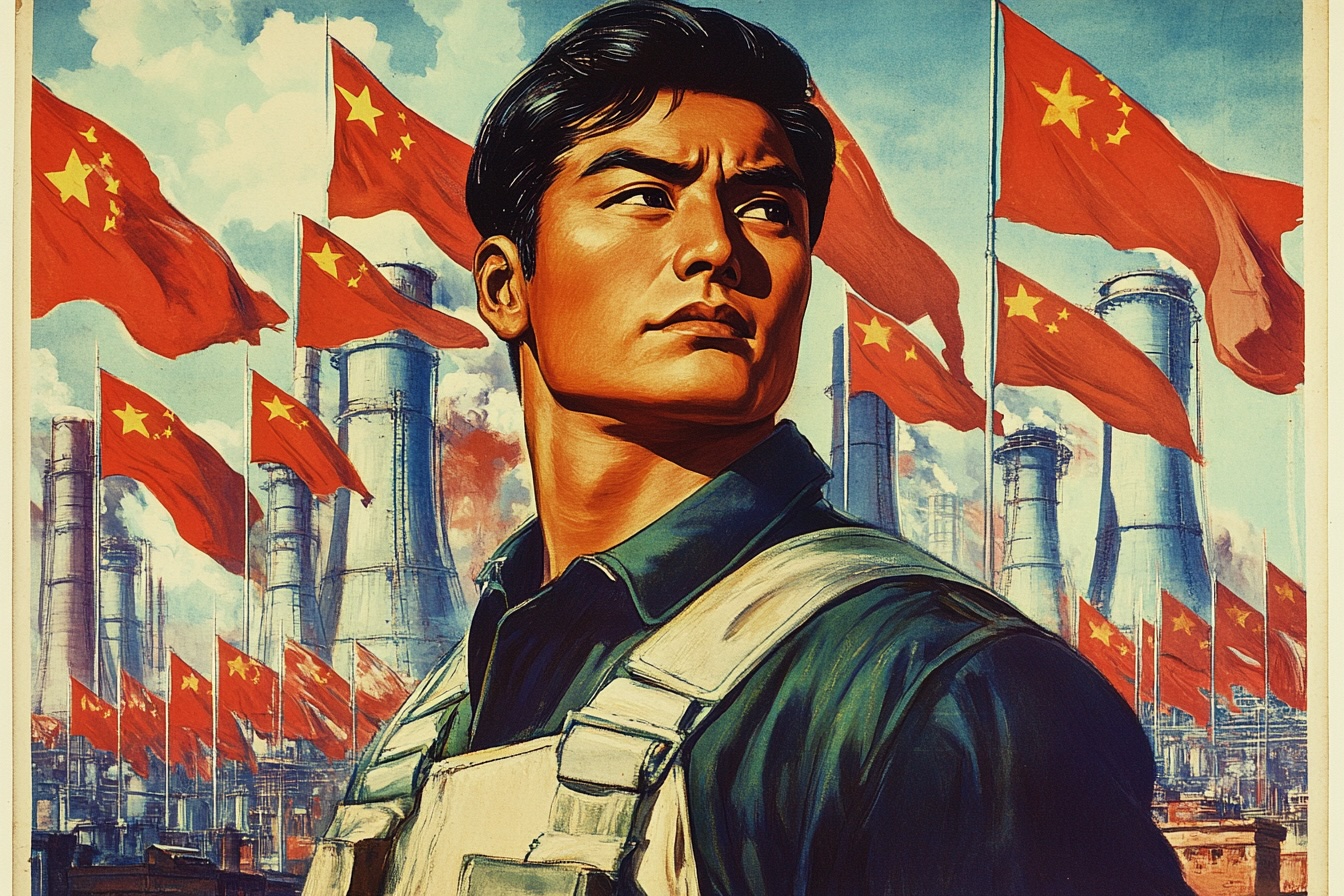
TL;DR
China's true AI competitive advantage lies in its mature commericalized and relatively inexpensive nuclear power capacity and building capacity. This advantage allows China to trade energy for access to advanced GPUs and AI compute resources from countries that can't keep pace with the power demands of AI. This maybe is the only chance China could still be relevant in the AI race.
In the world of artificial intelligence, data and computational power are the twin engines driving innovation. While most of the conversation revolves around algorithms, models, and hardware, there's a hidden force that often gets overlooked—energy. AI models like OpenAI's GPT-4 require massive amounts of energy for training, and it turns out that China's real competitive edge might lie not in silicon, but in something much more elemental: power generation.
To grasp how crucial energy is, let's dive into some figures. Training a large language model like GPT-4, for example, requires an astounding 51,773 megawatt-hours (MWh) of energy—enough to power an average U.S. household for over 5,000 years. In traditional terms, this translates into running 10,000 Nvidia Tesla V100 GPUs at full throttle for 150 days straight.
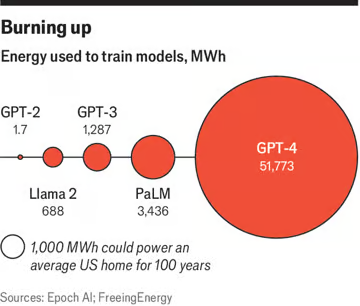
For nations that rely heavily on advanced AI development, the energy bill can be astronomical. This is where China’s underappreciated advantage comes into play: its nuclear energy capacity.
As of mid-2024, China boasts 56 operational nuclear reactors with a total installed capacity of 58,218 MWe. In just the first half of 2024, these reactors generated an impressive 212,261,000 MWh of electricity. For comparison, the energy required to train GPT-4 is a mere drop in this ocean, accounting for just 0.02% of China’s total nuclear output in six months.
But that’s not all. China has approved the construction of 11 more reactors, each with a 1,000 MWe capacity, which will add another 85,628,220 MWh of energy annually. In short, China is on track to comfortably power the training of multiple GPT-4-like models each year—without breaking a sweat.
In the AI race, computational power—measured in FLOP/s (Floating Point Operations per Second)—is the metric everyone obsesses over. But here's the kicker: to convert FLOP/s into tangible AI capabilities, you need energy. A lot of it.
Countries with abundant computational power but insufficient energy face a bottleneck. Meanwhile, China, which is still grappling with restrictions on high-end semiconductor technology, has an untapped reservoir of energy that could redefine its AI strategy. While the U.S. dominates on the chip side of things, China is quietly becoming an AI energy superpower.
Nvidia’s Tesla V100, one of the leading GPUs for AI, consumes about 300W of power. When scaled to the levels needed for training models like GPT-4, the energy requirement becomes a significant factor. For countries running massive GPU clusters, the power bill can quickly spiral out of control. This presents a unique opportunity for China.
While the U.S. may lead in computational horsepower, many countries face a crucial shortfall in energy. And that’s where China’s nuclear reactors come into play. Imagine a scenario where China, leveraging its abundant and inexpensive nuclear power, could trade energy credits for access to advanced GPUs and AI compute resources from countries that can’t keep pace with the power demands of AI.
A new strategy emerges: by using its nuclear power capacity as a bargaining chip, China could position itself as an indispensable energy supplier to AI-driven economies. Instead of competing in chip production, China could provide the fuel—energy—to power AI around the world.
In an era where the U.S. sanctions are making it increasingly difficult for China to access cutting-edge chips and GPUs, China could turn the tables by treating energy as currency. Instead of relying on domestic chip production (which is currently hampered by a lack of EUV lithography machines from ASML), China could strike deals with countries that have access to high-end computing resources but lack the power infrastructure to keep them running efficiently.
By using energy to barter for compute resources, China could remain a major player in the AI race without needing to directly compete with the U.S. in semiconductor manufacturing. This strategy not only helps China bypass the sanctions but also creates a new economic paradigm where energy—not just chips—becomes a key currency in the AI world.
As we look to the future, it’s clear that the AI competition will be shaped by more than just silicon. Energy availability and consumption will be the defining factors in scaling up AI capabilities. With its unmatched nuclear power capacity, China might not need the most advanced chips to stay relevant in the AI game—it just needs to power the world’s most energy-hungry AI models.
Country/Region |
Nuclear Power Plant Model |
Construction Start |
Expected Grid Connection |
Cost (USD/kW) |
Construction Period (years) |
USA |
AP1000 (Vogtle Units 3 & 4) |
2013 |
2023 |
>12000 |
10 |
USA |
AP1000 (Subsequent Projects) |
-- |
-- |
8000 (5000 in batch) |
-- |
Finland |
EPR (Olkiluoto Unit 3) |
2005 |
2022 |
6750 |
17 |
France |
EPR (Flamanville Unit 3) |
2007 |
2023 |
7700 |
16 |
UK |
EPR (Hinkley Point C Units 1 & 2) |
2018/2019 |
2027/2028 |
11100 |
10 |
India |
VVER-1200 (Kudankulam Units 3-6) |
2017/2021 |
2023/2027 |
5200 |
6 |
China |
AP1000 (First Unit) |
-- |
-- |
1/4 of US cost ~ 2500 |
-- |
China |
AP1000 (Batch Production) |
-- |
-- |
1/2.5-1/3 of US cost ~ 4000 to 3000 |
-- |
If China could build AP1000 reactors in a batch, the cost would be 1/2.5 ~ 1/3 of the US, and the time would be 1/3 of the US, roughly around 3000 ~ 4000 USD/kW.
The training time of GPT-4 is around five to six months. So, 10,000 V100, running for 150 days on full power, means energy consumption of 7200000000 watt-hours, or 7,200 MWh.
China's new reactor’s average capacity is 1,000 MWe. Assuming a capacity factor of 88.85%, the reactor operates at this level throughout the year.
\[\begin{align*}
\text{Annual energy output} &= 1,000 \, \text{MWe} \times 88.85\% \times 24 \times 365 \\[10pt]
\text{Annual energy output} &= 1,000 \times 0.8885 \times 8,760 \, \text{hours} = 7,780,860 \, \text{MWh/year}
\end{align*}\]
So, one new reactor would generate 7,780,860 MWh per year.
- Energy Required to Train One GPT-4 Model
The energy required to train one GPT-4 model is 51,773 MWh.
- Calculate How Many GPT-4 Models Can Be Trained
Now, to calculate how many GPT-4 models could be trained with the annual energy output of one reactor:
\[\begin{align*}
\text{Number of GPT-4 models} &= \frac{7,780,860 \, \text{MWh/year}}{51,773 \, \text{MWh/GPT-4 model}} \\[10pt]
&= 150.24
\end{align*}\]
i.e. one reactor can train 150 GPT-4 models per year.
Insights🔥
And the current training cost of GPT-4 is around $63 million., let's say China's nuclear-generated power price is 1/3 of the US, then the cost would be $21 million.
Now, given U.S. sanctions blocking China's access to sub-7nm chips by cutting off advanced EUV machines from ASML and restricting Nvidia from selling top-tier GPUs, China’s best shot might be to focus on what it can do fast and cheaply—build nuclear reactors. Using "energy/power" as a credit system, China could trade its abundant energy for access to GPUs and computing resources from countries* that have the technology but are struggling with the massive energy consumption of AI training. This strategic pivot could keep China highly competitive in the AI race, even as it navigates the restrictions imposed by the U.S.-led Western bloc.
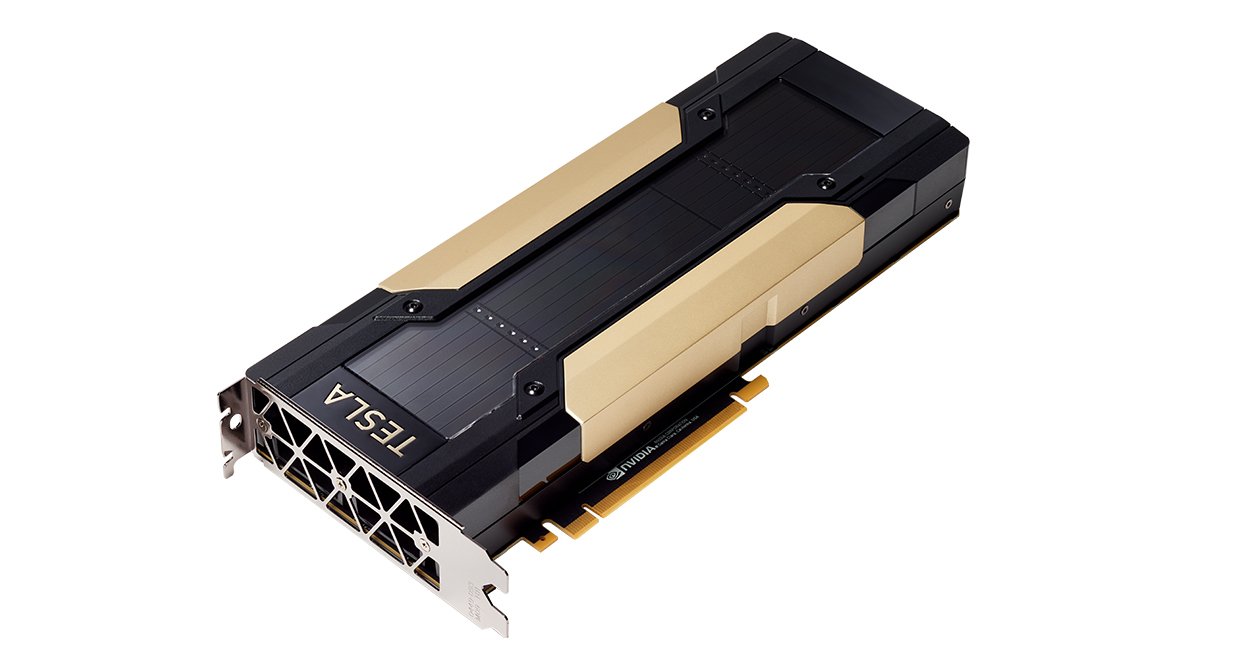
Countries could be potential trading partners
like Mid East, South Asia/ASEAN, Leading African countries, etc. Because NATO countries, Australia, New Zealand, Japan, South Korea and even Taiwan will and already are in the U.S. led camp. Not too many countries are left and not too much time left.